Elasticsearch
The heart of the free and open Elastic Stack
Elasticsearch is a distributed, RESTful search and analytics engine capable of addressing a growing number of use cases. As the heart of the Elastic Stack, it centrally stores your data for lightning fast search, fine‑tuned relevancy, and powerful analytics that scale with ease.
New to Elasticsearch? Get up and running in no time.
Watch videoLay a strong foundation for working with Elasticsearch in our Elasticsearch Engineer training.
View trainingBuild advanced Elasticsearch skills for tuning relevance, text analysis, and more.
View trainingDive deeper into the new Elasticsearch Relevance Engine
The Elasticsearch Relevance Engine™ (ESRE) is designed to power artificial intelligence-based search applications. Use ESRE to apply semantic search with superior relevance out of the box (without domain adaptation), integrate with external large language models (LLMs), implement hybrid search, and use third-party or your own transformer models.
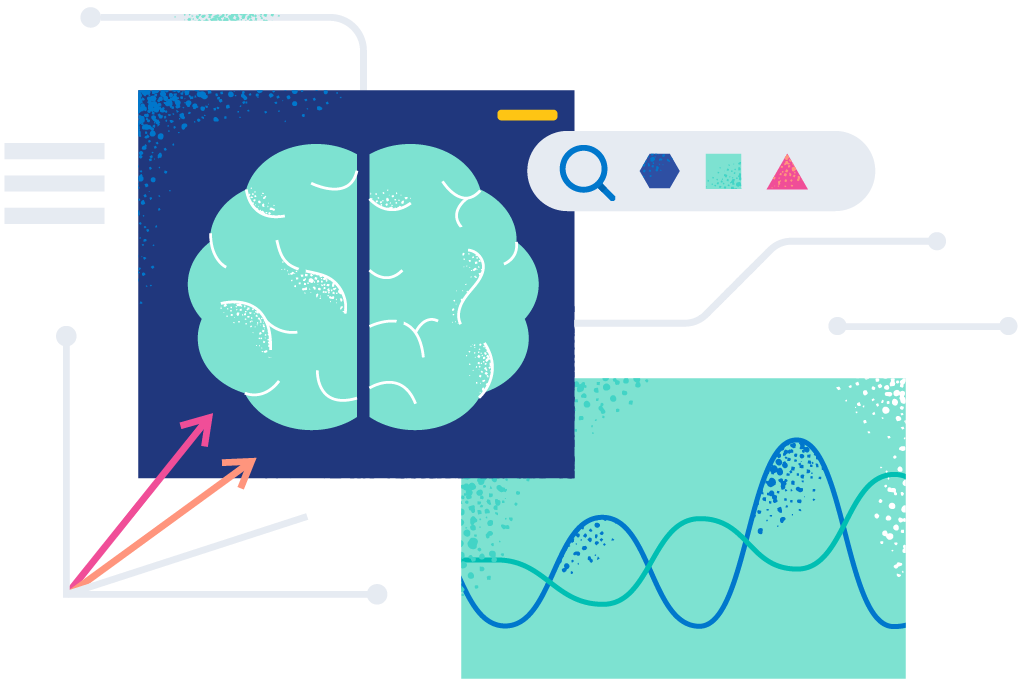
Scalability
Run it on your laptop. Or hundreds of servers with petabytes of data.
Go from prototype to production seamlessly; you talk to Elasticsearch running on a single node the same way you would in a 300-node cluster.
It scales horizontally to handle kajillions of events per second, while automatically managing how indices and queries are distributed across the cluster for oh-so-smooth operations.
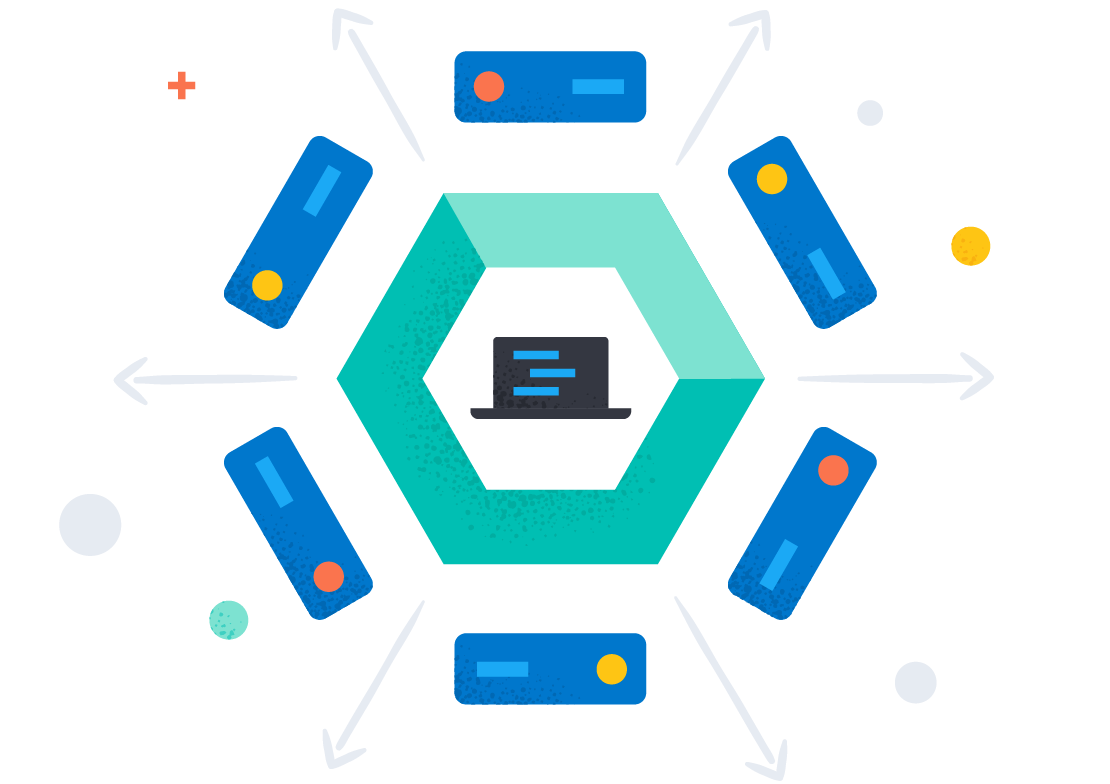
Resiliency
We cover the bases while you swing for the fences.
Hardware rebels. Networks partition. Elasticsearch detects failures to keep your cluster (and your data) safe and available. With cross-cluster replication, a secondary cluster can spring into action as a hot backup. Elasticsearch operates in a distributed environment designed from the ground up for perpetual peace of mind.
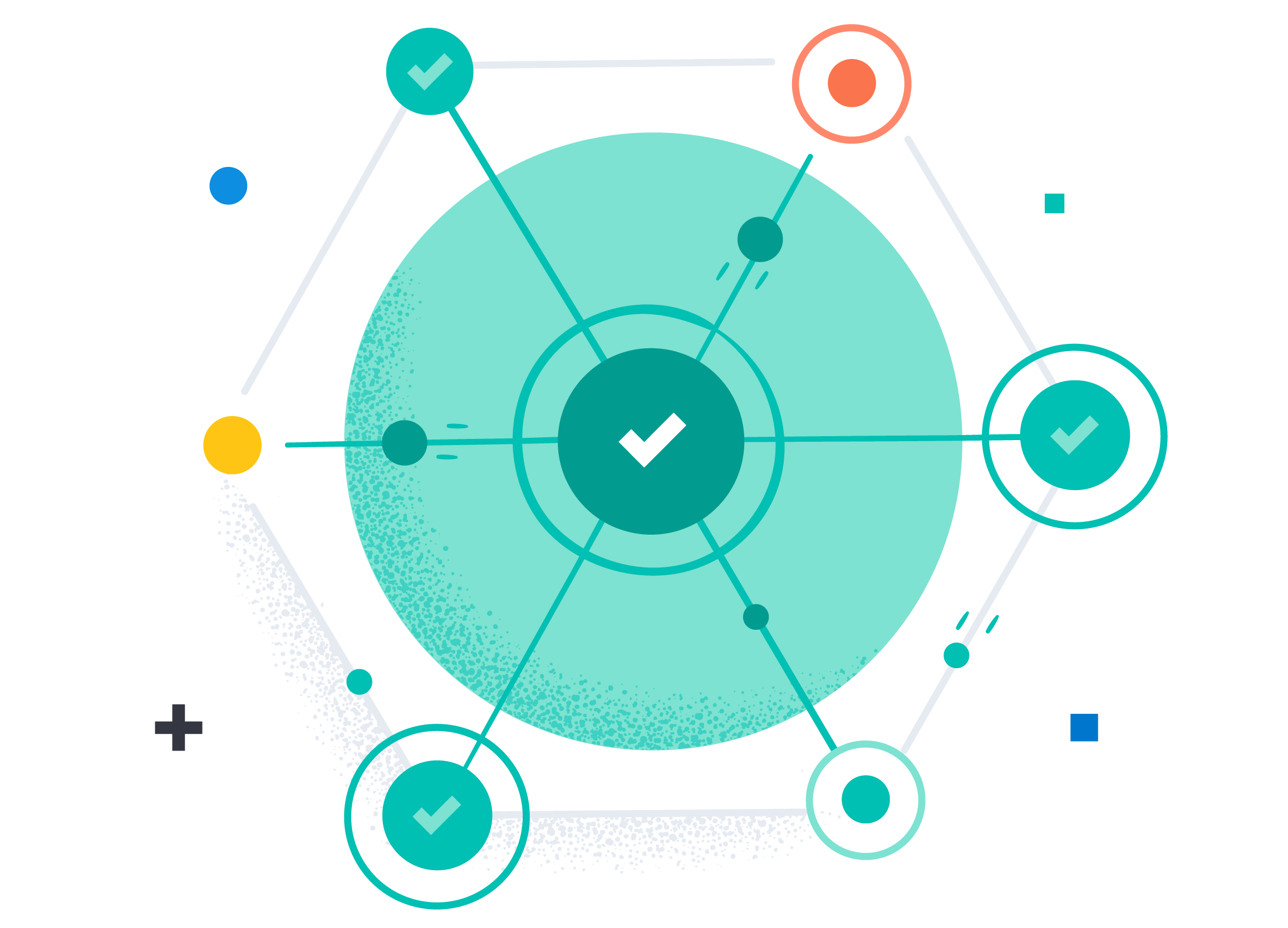
FLEXIBILITY
Store and explore data to fit your needs.
Data is constantly evolving, and it can become expensive to store and search all of it. With Elasticsearch you can balance performance and cost. Store data locally for fast queries or remotely on low-cost S3 for unlimited data. With runtime fields, you can also quickly onboard your data — and adapt to changes.
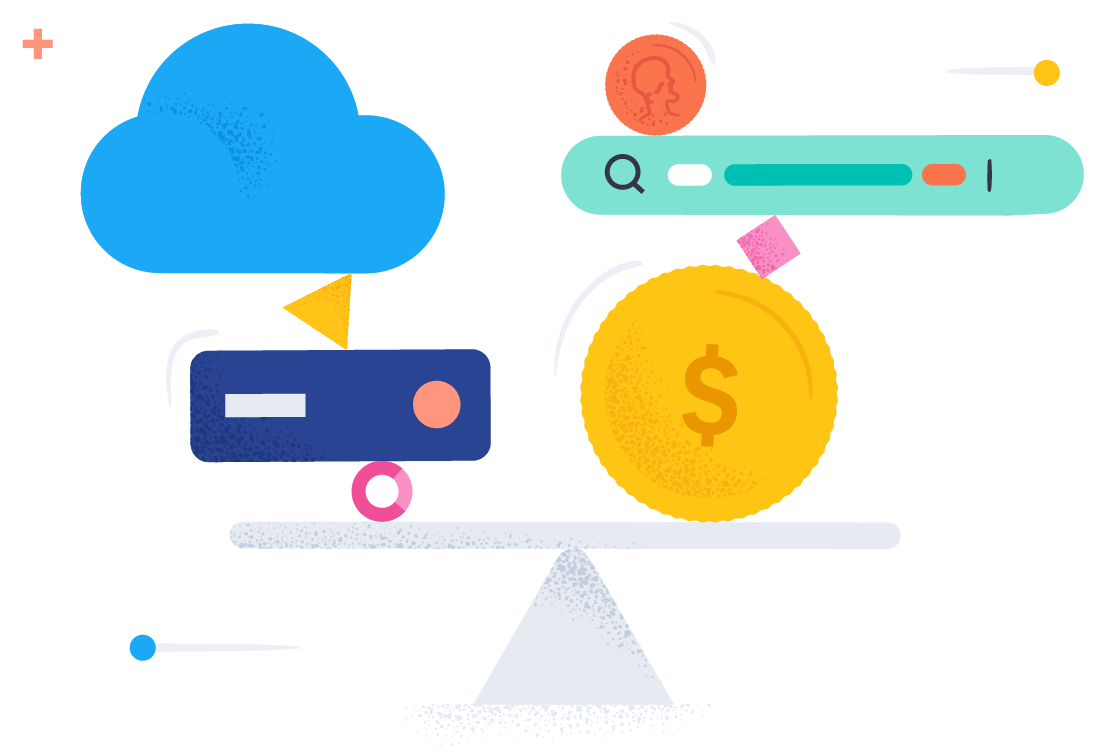
Distribution
Deploy your way
Wherever your search takes you, we'll be there.
Elastic Cloud
Deploy hosted Elasticsearch and Kibana on AWS, Google Cloud, and Azure
Spin up a fully loaded deployment on the cloud provider you choose. As the company behind Elasticsearch, we bring our features and support to your Elastic clusters in the cloud.
On-Prem
Download Elasticsearch
Grab a fresh installation and start running Elasticsearch on your machine in just a few steps.
Client Libraries
Interact with Elasticsearch in the programming language you choose
Elasticsearch uses standard RESTful APIs and JSON. We also build and maintain clients in many languages such as Java, Python, .NET, SQL, and PHP. Plus, our community has contributed many more. They're easy to work with, feel natural to use, and, just like Elasticsearch, don't limit what you might want to do with them.