Fraud in financial services: Leaning on generative AI to protect a rapidly expanding attack surface
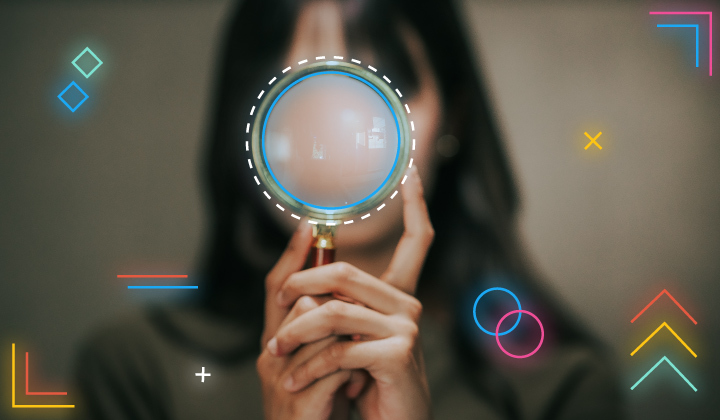
The financial ecosystem is experiencing an exciting evolution in global payment systems, driven by a digital first approach. The pandemic changed both consumer and business behavior toward adopting new and emerging forms of digital channels. The increased use of digital wallets, real-time payments, embedded payments (such as ApplePay and GooglePay), peer to peer payment systems, and other emerging vehicles ramped up. In fact, according to statista.com, digital payments are projected to reach $11.55 trillion in transaction value within 2024 — all while cash as a form of payment continues to decrease globally.
While this revolution has greatly improved efficiency and reduced friction for customers, banks, and merchants, it has also resulted in an exponential growth in fraud in financial services, within the global digital payments market. The TransUnion 2023 State of Omnichannel Fraud Report found that digital fraud continues to rise, with 4.6% of all customers’ digital transactions globally suspected to be fraudulent. Fraud attempts overall have increased by 80% from 2019 to 2022. This is compounded by the fact that fraudsters have most recently commercialized generative AI and LLM technology, which has enabled them to attack with greater sophistication — for example, writing better, more convincing phishing emails with a higher rate of impact than we have ever seen in the past.
With AI and generative AI now being leveraged as a weapon for fraudsters, the new real requirement is for FSIs to increase their own defensive use, deployment, training, and evolution of AI to stop fraud. FSIs are thus looking to trusted vendors as an important and versatile partner to protect our entire global financial infrastructure.
How are artificial intelligence and generative AI transforming financial fraud detection?
AI technologies, particularly machine learning, have been used for many years within finance to execute high speed trading, detect fraud, improve risk scoring, and deliver overall efficiencies. However, we are now seeing a rapid adoption of AI/ML for monitoring and detecting, along with responding to fraud across the growing attack surface. Most recently, generative AI is being deployed to increase the impact of a fraud analyst in really amazing ways, such as:
Anomaly detection
One of the key strengths of AI in fraud detection lies in its ability to detect anomalies or deviations from normal patterns of behavior. Machine learning algorithms can learn from historical data to establish a baseline of normal behavior for each account or transaction type. Any deviation from this baseline can then trigger an alert for further investigation, potentially flagging fraudulent activity that might have gone unnoticed by human analysts. The emergence of generative AI has further improved anomaly detection — by learning the underlying structure of genuine transactions, generative models can detect subtle deviations indicative of fraudulent activity, complementing traditional anomaly detection methods.
Behavioral analysis
AI-powered fraud detection systems can also analyze user behavior across multiple channels and touchpoints to identify suspicious activities. Know Your Customer (KYC) is an entirely new way of managing this risk. By monitoring factors such as transaction frequency, location, device type, and interaction patterns, these systems can detect anomalies that may indicate fraudulent behavior, such as unauthorized account access or unusual spending patterns.
Ecosystem analysis
Another valuable application of AI in fraud detection is the analysis of networks and ecosystems, which involves examining the relationships and connections between entities (e.g., customers, accounts, transactions). Systems supported by AI and generative AI can uncover complex fraud networks and identify previously undetected links between seemingly unrelated entities, facilitating the detection and disruption of organized fraud rings.
Priority use cases in FSI
The deployment of AI in fraud detection and prevention is already yielding tangible results across the financial services industry. Leading banks, payment processors, and fintech companies are leveraging AI-powered solutions to detect and prevent fraud in real time, protecting both their customers and their bottom line. For example:
Payment fraud prevention: AI algorithms can analyze transaction data in milliseconds to identify fraudulent transactions, such as unauthorized purchases or account takeovers, and block them before they are completed.
Identity verification: AI-powered biometric authentication systems can accurately verify users’ identities based on facial recognition, voice recognition, or behavioral biometrics, reducing the risk of identity theft and account fraud.
Anti-money laundering (AML) compliance: AI systems can analyze vast amounts of transaction data to identify suspicious patterns indicative of money laundering or other illicit activities, helping financial institutions comply with AML regulations and mitigate regulatory risk.
Ethical and regulatory considerations
While the benefits of AI in fraud detection are undeniable, it is essential to address ethical and regulatory considerations to ensure responsible and equitable use of these technologies. AI algorithms should be transparent and explainable, enabling stakeholders to understand how decisions are made and identify potential biases or errors. Also of course, while AI can automate many aspects of fraud detection, human oversight remains critical to review flagged cases, investigate suspicious activities, and make informed decisions based on contextual information that AI systems may lack.
However, one area of increasing concern surrounds data privacy and security. Financial institutions must safeguard customer data and ensure compliance with data protection regulations to maintain trust and confidence in AI-powered fraud detection systems. As FSIs incorporate third parties into the fraud process (for example, in the case of generative AI), combining public information with proprietary data naturally raises privacy and regulatory concerns. Safeguarding their systems with the right tools is thus imperative.
The role of Elasticsearch in AI, generative AI, and financial fraud detection
At its core, fraud is undoubtedly a data problem. As financial institutions continue to innovate in the realm of fraud detection and prevention, the role of Elasticsearch®, an open-source distributed search and analytics engine, becomes increasingly significant. Elasticsearch's scalability, real-time data processing capabilities, and advanced search functionalities make it a valuable asset in the fight against fraud in financial services.
Real-time data analysis: Elasticsearch's distributed architecture allows financial institutions to ingest, index, and analyze vast volumes of transactional data in real time, across time and space (including geospatial analysis), enabling them to detect and respond to fraudulent activities as they occur.
Complex querying and analysis: With Elasticsearch, fraud analysts can perform complex queries, aggregations, and machine learning-driven analytics on large data sets with ease. By leveraging Elasticsearch's advanced search and analytics features, financial institutions can uncover hidden insights and correlations that may signal fraudulent behavior.
Scalable infrastructure: Elasticsearch's scalable infrastructure ensures that financial institutions can handle the growing volume and complexity of data associated with fraud detection and prevention efforts. Whether analyzing millions of transactions or conducting network analysis to uncover fraudulent schemes, Elasticsearch provides the necessary scalability and performance to support these critical tasks.
Gen AI: The Elasticsearch Relevance Engine (ESRE) is a powerful tool that combines artificial intelligence and text search capabilities to create smarter generative AI applications in financial services. With ESRE, developers can make search results more accurate and relevant by using advanced algorithms that integrate with large language models for generative AI search engines. Elastic AI Assistants can enable faster investigations, reduce time to resolution, and enable every analyst to have a digital partner to help guide them quickly and completely through the remediation process.
Data privacy and security: In highly regulated industries like financial services, ensuring data privacy and security is crucial. Elastic® includes native support for role-based and attribute-based access control to ensure that only those roles with access to data can see it, even for chat and question answering applications. Elasticsearch can support your organization’s need to keep certain documents accessible to privileged individuals, helping your organization to maintain universal privacy and access controls across all of your search applications.
The future of fraud, AI, and generative AI . . .
The role of generative AI in financial fraud detection and prevention is transformative, offering financial institutions the ability to combat fraudulent activities with unprecedented speed, accuracy, and efficiency. However, to realize the full potential of AI-powered fraud detection, financial institutions must also leverage the right tools and technologies, such as Elasticsearch, to effectively analyze, interpret, and respond to fraud-related data. With continued innovation and collaboration, generative AI and Elasticsearch will undoubtedly remain integral tools in preventing fraud in financial services, safeguarding the integrity of the financial system and protecting the interests of customers and FSIs alike.
Learn more about how to accelerate fraud detection and prevention with Elastic.
The release and timing of any features or functionality described in this post remain at Elastic's sole discretion. Any features or functionality not currently available may not be delivered on time or at all.
In this blog post, we may have used or referred to third party generative AI tools, which are owned and operated by their respective owners. Elastic does not have any control over the third party tools and we have no responsibility or liability for their content, operation or use, nor for any loss or damage that may arise from your use of such tools. Please exercise caution when using AI tools with personal, sensitive or confidential information. Any data you submit may be used for AI training or other purposes. There is no guarantee that information you provide will be kept secure or confidential. You should familiarize yourself with the privacy practices and terms of use of any generative AI tools prior to use.
Elastic, Elasticsearch, ESRE, Elasticsearch Relevance Engine and associated marks are trademarks, logos or registered trademarks of Elasticsearch N.V. in the United States and other countries. All other company and product names are trademarks, logos or registered trademarks of their respective owners.