Generative AI in financial services: The role of the Elasticsearch Relevance Engine
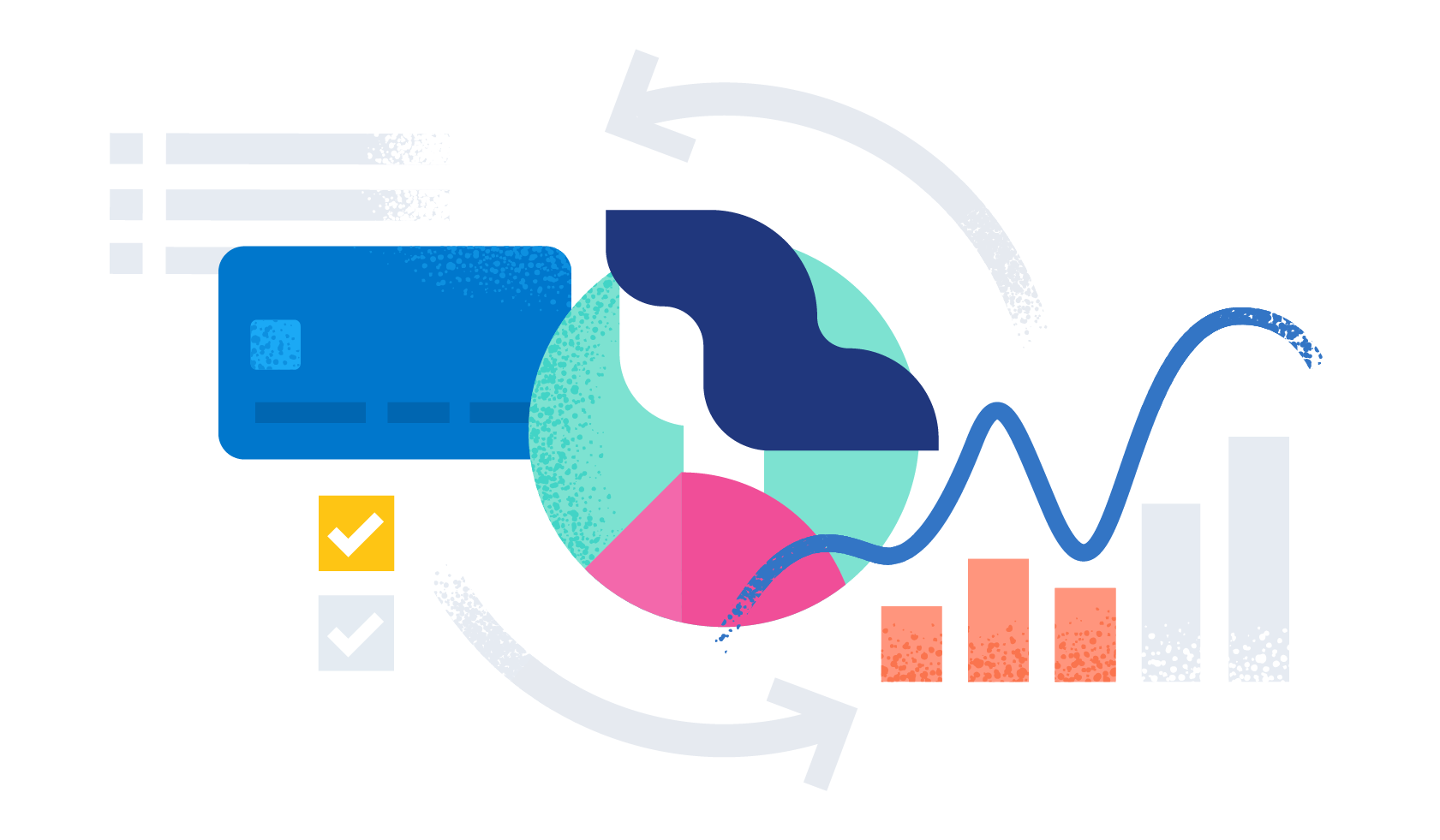
Generative artificial intelligence (GAI) is undoubtedly one of the biggest trends across industries in 2023. In a recent survey, almost two-thirds of executives believe generative AI will have a high or extremely high impact on their organization in the next three to five years. Executives anticipate spending the next 6–12 months focused on increasing their understanding of how generative AI works, evaluating internal capabilities, and investing in generative AI tools.
The financial services community adopted artificial intelligence & machine learning technology early on. AI largely gained prominence with the advent of high-frequency trading in the early 2000s, where financial institutions started using machine learning algorithms to identify patterns in market data and execute trades automatically, aiming to gain a competitive edge. Financial institutions have come a long way since then, implementing AI/ML across business functions, technology (e.g., AIOps), and cybersecurity. After all, detecting anomalies in data, and surfacing those insights in real time has a natural connection point with financial services, where market shifts, risks, and opportunities can present themselves at any point in time.
However, despite the many obvious use cases in financial services, the industry has fallen behind in applying AI, due to its highly regulated nature (among other legitimate factors). Some reports have placed the industry last in both current utilization of AI and future usage. Obtaining large and diverse data sets can be problematic in the financial industry, which makes it challenging to properly train models. Then of course, there are hesitancies (both real and cultural) where the industry would rather rely on human intervention across functions, versus depending on systems and machines. While these are challenges for the industry as a whole, it’s also a great opportunity for FSIs who successfully implement AI driven solutions to obtain a competitive advantage in the market.
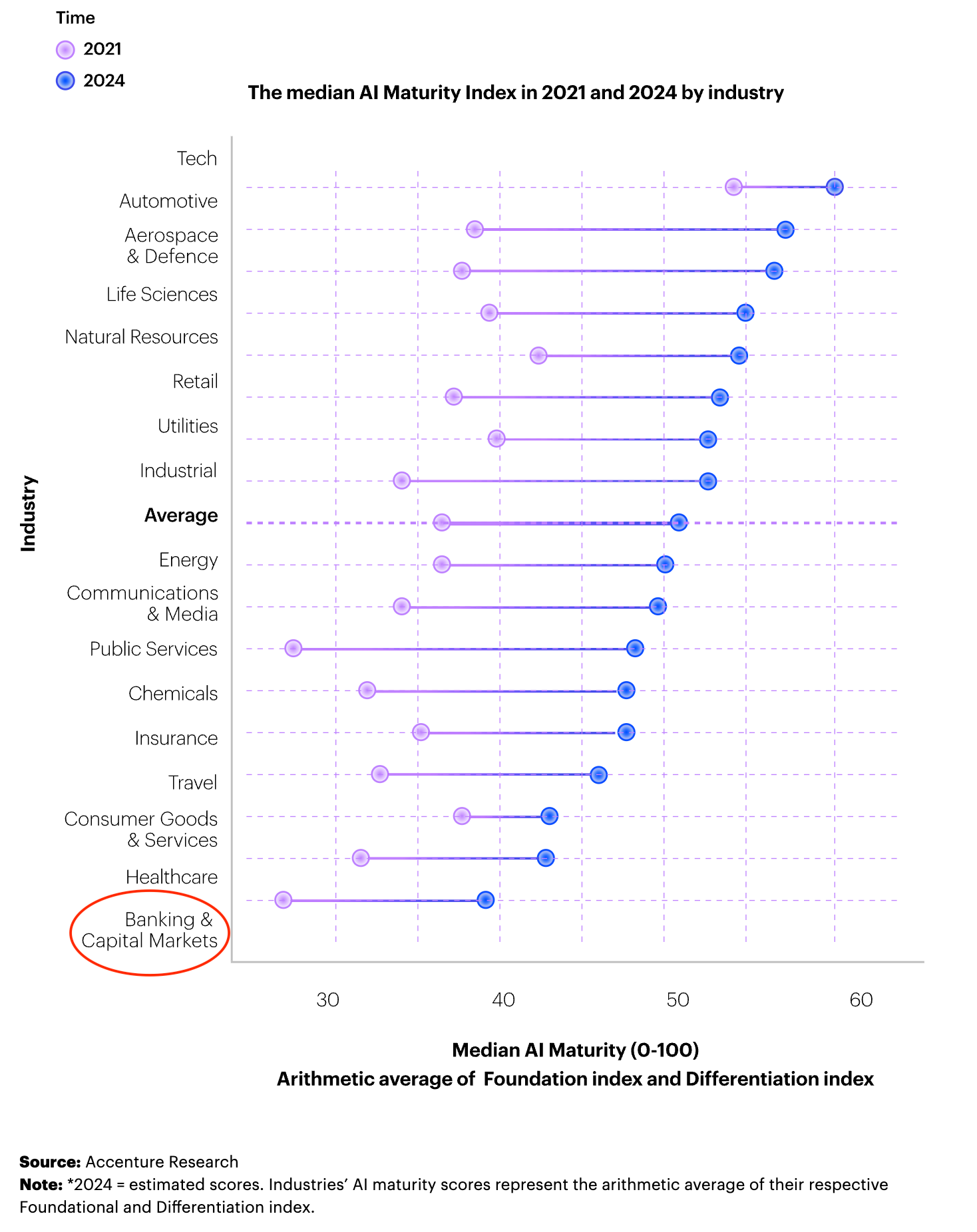
Can generative AI unlock the true potential of FSI use cases?
Generative AI presents a real opportunity for FSIs. It allows financial institutions to overcome data limitations, improve risk management, enhance fraud detection, personalize services, and refine investment strategies. By using generative models, financial institutions can create additional data points that expand the size and diversity of their data sets. This enables them to train more accurate and robust machine learning models, improving the quality of their predictions and decision-making processes.
As the industry grapples with which use cases to tackle first, the decision ultimately will be guided by which “jobs” serve the highest interest of customers and provide the greatest commercial benefit to providers. Some of these include:
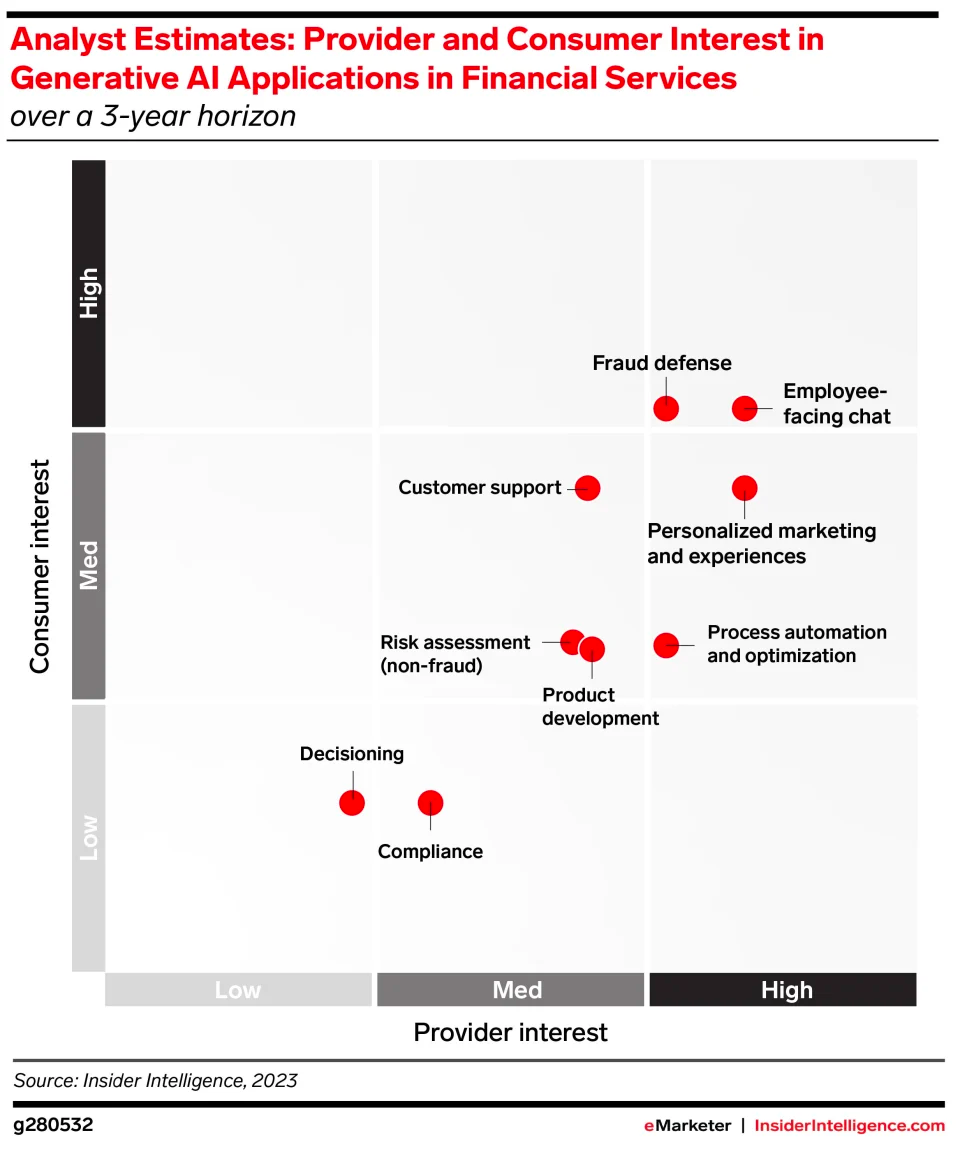
Customer support and employee chat: GAI can revolutionize customer support in financial services by automating processes, understanding customer needs, and providing efficient assistance, ultimately reducing the workload on support teams and improving overall service quality. Chatbots can assist customers (and employees) with account inquiries, transaction history, loan applications, and more to retrieve the required information in real time.
Fraud detection: Generative AI techniques can contribute to identifying fraudulent activities and detecting anomalies in financial transactions. By learning patterns from existing data, generative models can create new data points that conform to the learned patterns. Financial institutions can then compare real-time transactions against the generated patterns, enabling the detection of unusual or fraudulent behavior that might go unnoticed with traditional rule-based systems.
Personalized marketing and experiences: Generative AI can enhance the customer experience in financial services by creating personalized recommendations, customized reports, or targeted marketing campaigns. By analyzing customer data and generating tailored content or offers, financial institutions can better meet individual customer needs, improve engagement, and increase customer satisfaction.
Risk and compliance: GAI can identify patterns, trends, and anomalies that may indicate potential risks. This can include analyzing market data, customer behavior, credit histories, and other relevant information. Also, by automating the monitoring of transactions, communications, and other activities, it is possible to identify potential compliance breaches and flag suspicious activities.
Pushing GAI past its limitations
While GAI has shown impressive capabilities in generating content, conversations, and images, for the use cases above (and others), generative AI still has its limitations. GAI’s relevance is largely dependent on the training data it has been exposed to. When GAI is trained solely on publicly available data from the internet, its generated answers may have limited scope and can be prone to presenting incorrect information as accurate, often referred to as "hallucinations." Also, for the above use cases, a financial institution would need to combine public information with proprietary data to find relevant information. But this naturally raises privacy and regulatory concerns, especially when a company's proprietary data is exposed to third party technology.
To make the most of GAI in financial services, it is essential to have a unified data platform where the organization's data is stored, making it easily (and safely) accessible and searchable in one centralized location. This prerequisite allows for the seamless integration of domain-specific context from the organization's data, thereby enhancing the performance and value of GAI for achieving objectives in the financial services industry.
In order to drive this, Elastic® recently launched the Elasticsearch Relevance EngineTM (ESRETM), a powerful tool that combines artificial intelligence and text search capabilities to create smarter search applications. With ESRE, developers can make search results more accurate and relevant by using advanced algorithms that integrate with large language models for generative AI search engines. ESRE provides features like advanced ranking, the ability to understand and process natural language, and integration with models like GPT-3 and GPT-4 for summarizing content. ESRE also addresses challenges such as privacy, scalability, and cost, making it easier for financial institutions to create search experiences that understand user intent and deliver more meaningful results. The outcomes include:
Better quality (and more) training data: GAI's performance heavily relies on the quality and diversity of the training data. If the training data is insufficient or biased, GAI may produce inaccurate or biased outputs. Elastic can help overcome this limitation by providing access to a vast amount of data through its AI-backed search capabilities. Financial institutions can leverage their own internal data, which is often more relevant and reliable, to enhance the accuracy and relevance of GAI's output.
Improved contextual understanding: GAI may struggle to fully grasp the context and nuances of specific financial services domains. Elastic can address this limitation by integrating GAI with its search capabilities, enabling organizations to extract domain-specific context from their internal data. By combining GAI with the comprehensive knowledge captured in the organization's data, Elastic enhances the understanding of GAI and ensures more accurate and contextually relevant responses.
Focus on data privacy and security: GAI typically requires large amounts of data to achieve optimal performance. However, in highly regulated industries like financial services, ensuring data privacy and security is crucial. Elastic includes native support for role-based and attribute-based access control to ensure that only those roles with access to data can see it, even for chat and question answering applications. Elasticsearch® can support your organization’s need to keep certain documents accessible to privileged individuals, helping your organization to maintain universal privacy and access controls across all of your search applications.
With ESRE, financial institutions can enhance their search capabilities to deliver more accurate and relevant results to their customers and employees, while ensuring sensitive financial data remains protected. In an industry where finding and accessing the right information at speed is crucial, ESRE shines.
In this blog post, we may have used or referred to third party generative AI tools, which are owned and operated by their respective owners. Elastic does not have any control over the third party tools and we have no responsibility or liability for their content, operation or use, nor for any loss or damage that may arise from your use of such tools. Please exercise caution when using AI tools with personal, sensitive or confidential information. Any data you submit may be used for AI training or other purposes. There is no guarantee that information you provide will be kept secure or confidential. You should familiarize yourself with the privacy practices and terms of use of any generative AI tools prior to use.
Elastic, Elasticsearch, ESRE, Elasticsearch Relevance Engine and associated marks are trademarks, logos or registered trademarks of Elasticsearch N.V. in the United States and other countries. All other company and product names are trademarks, logos or registered trademarks of their respective owners.