What is AIOps? A beginner's guide
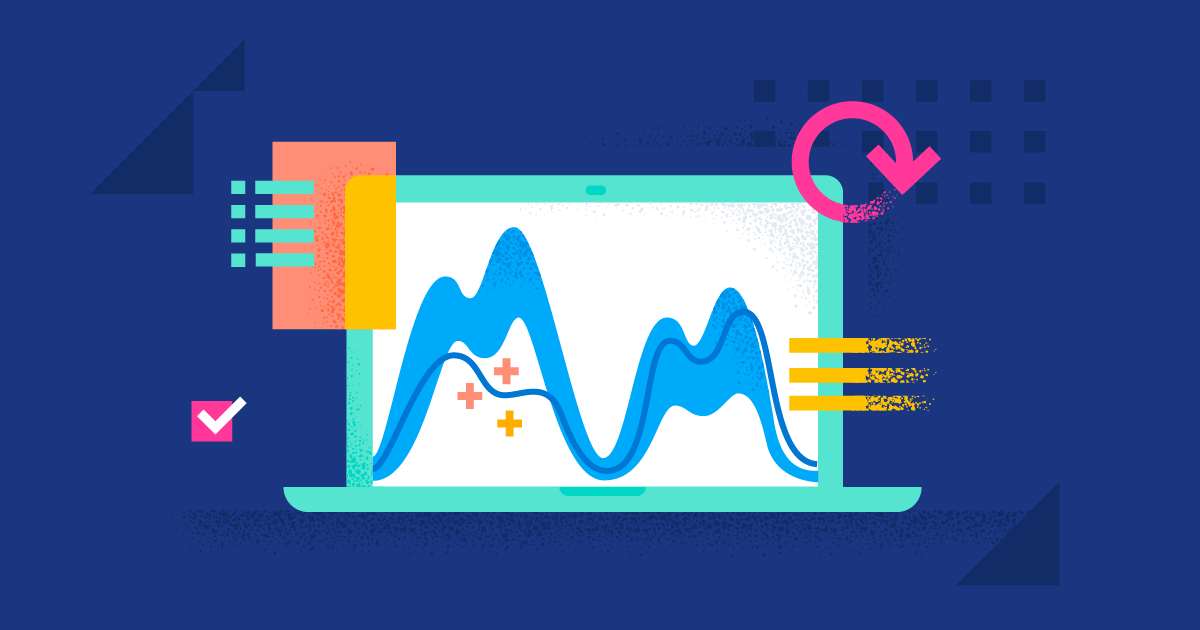
Understanding AIOps for observability
Artificial Intelligence for IT Operations (or AIOps for short) continues to be a hot topic among developers, SREs, and DevOps professionals. The case for AIOps is especially crucial given the expansive nature of today’s observability efforts across hybrid and multi-cloud environments. As with most observability platforms, it all starts with your telemetry data: metrics, logs, traces, and events.
Once IT operations teams collect and begin analyzing that data, the benefit of AIOps becomes rapidly clear. AIOps aims to accurately and proactively identify areas that need attention and assist IT teams in solving issues faster. As human beings, we cannot keep up with analyzing petabytes of raw observability data. Adding AIOps delivers a layer of intelligence via analytics and automation to help reduce overhead for a team. Let's dive in to answer common questions on this critical topic!
What is AIOps and how can it help me?
Simply put, AIOps is the ability of software systems to ease and assist IT operations via the use of AI/ML and related analytical technologies. AIOps capabilities can be applied to ingestion and processing of various operational data, including log data, traces, metrics, and much more.
Definitions and explanations by Gartner™, Forrester™, and others seek to clarify the often murky and confusing world of AIOps. AIOps can help significantly reduce the time and effort to detect, understand, investigate, determine root cause, and remediate issues and incidents faster. Saving time during troubleshooting can, in turn, help IT personnel focus more of their energy on higher-value tasks and projects.
A search toolkit for the AI era
The Elasticsearch Relevance Engine (ESRE) gives developers the tools they need to build AI-powered search apps.
Build Generative AI Search Engines and ApplicationsWhy do you need AIOps as part of your observability strategy?
From digital transformation initiatives to cloud migration to distributed, hybrid, or cloud-native application deployments, market dynamics are dramatically changing the IT operations landscape.
The landscape changes have the following three characteristics:
- Data volume: Volume of data for observability continues to increase exponentially
- Complexity: Applications, workloads, and deployments continue to become more complex, ephemeral, and distributed
- Pace of change: Rate at which changes (application and infrastructure) occur is faster than ever before
These are not mutually exclusive. In some ways, quite the opposite. For example, high rates of change and complex deployments utilizing auto-scaling means an even higher volume of data. This increasing complexity means that humans will increasingly depend on systems and automation to keep up with the changes. And AIOps plays a key role in responding to these challenges.
Leveraging AI/ML to summarize and roll up data, and to intelligently tier the data for storage can help alleviate some of the volume challenges. Clear visual depictions of an application environment, via infrastructure and service dependency maps for example, and contextual navigation help align troubleshooting efforts with how users naturally think of their deployment. Furthermore, auto-surfacing of problems and root cause analyses will address some of the other complexity challenges.
Observability products will need to keep track of all application and infrastructure changes and correlate those changes with system behavior and user experience, because change is often the root cause of acute, anomalous behavior. An upgrade or patch for a new feature with unintended consequences is a typical example. Enabling those correlations helps teams be more agile and adept at keeping pace with those frequent changes helping sustain service performance.
Thus AIOps plays a key role and, when implemented and used properly, can help navigate these challenges effectively, freeing up operations teams to focus on more important work.
Which observability use cases are best supported by AIOps?
Several observability workflows and use cases are already very well served with the application of AIOps techniques and technologies, for example:
- Service degradation such as sudden or unexpected variations in latency can be detected via anomaly detection.
- Massive volumes of data, such as unstructured or semi structured log messages, can be automatically classified, categorized, and summarized to help ease consumption and analysis.
- Multiple symptoms, events, and issues can be correlated to help cut down alert "noise" as well as reduce time to root cause determination.
- Automatic health scoring based on assessment of impact, extent of anomalies, and other measures help surface the most critical issues first, further reducing noise.
In the more well understood and time-tested "if this is the symptom then this is the likely root cause" relationships, AIOps can help automatically look for, detect, and classify those symptoms, and surface the potential root causes. AIOps can also enable remediation actions to fix routine or trivial issues. In a future blog, we will dive deeper into key use cases and how you can identify scenarios to apply AIOps in your day-to-day operations.
How does AIOps drive business value for an organization?
As with many initiatives in IT and software development, AIOps benefits an organization and team in multiple ways. While AIOps can significantly reduce the mundane and repetitive work required by IT operations (ITOps), site reliability engineering (SRE), and DevOps teams, there are also significant business benefits:
- Reducing MTTD, MTTR (mean time to detection and mean time to resolution) means less service downtime, improved SLAs, and better customer experience.
- Helping organizations deal with rapidly growing data volumes intelligently, reducing total cost-of-ownership (TCO), and alleviating scale challenges.
- Reducing signal and alert noise and implementing better automation helps free operations teams to take on higher-value initiatives.
- Improving an organization's ability to handle ever-increasing IT complexity and the overall pace of change, AIOps allows businesses to bring value to customers more quickly and frequently.
Given the volume, complexity, and pace of change in today's cloud-native and hybrid application environments, AIOps is increasingly moving from being a nice-to-have capability to a mission-critical competency for IT operations teams.
How do you build trust in AIOps and make it production-ready?
IT personnel, SREs, and DevOps engineers have a couple of adoption hurdles they must cross to successfully adopt and use AIOps for their observability use cases.
On the one hand, there are significant buzzword challenges, and the market for AIOps has a lot of buzzwords. Users are faced with questions such as what is the business value beyond those buzzwords? And whether AIOps will help them detect and remediate problems better and more efficiently than their current monitoring or observability setup. Beyond the buzzwords and hype, users may not always know if they will benefit from AI/ML for a specific use case.
And then there are trust hurdles. One such hurdle is users' inability to tell whether the AIOps based insights are accurate. Users might not even be aware of how comprehensive the analysis is, the information used, how the algorithms work, how conclusions are arrived at, or if those conclusions are relevant to their current investigation, resulting in general distrust of black box AIOps systems. In some cases organizational pressures or policies motivated by a lack of trust may also present barriers to AIOps adoption.
Our experience has shown that the best way for AIOps to provide its value is through its slow and steady adoption. First, identify specific, time-tested, and proven use cases to start adopting AIOps as a proof of concept (POC). Next, enable AIOps functionality on a smaller subset of your deployment while validating and socializing benefits and outcomes at each stage. Once you've seen some success, incrementally enable more AIOps functionality with a move towards production environments. This deliberate deployment path alleviates some of the traditional challenges associated with deploying new technology that can otherwise deter widespread AIOps adoption.
Testing and proving technology effectiveness in a smaller lab or non-production environment and measuring and showcasing results to management can help increase confidence and get buy-in before deploying AIOps in a real-world production environment. Such testing might unearth other gaps and requirements, such as missing or inconsistent data, shallow coverage, or insufficient storage or compute. As you deploy AIOps in production, check to see if your Observability solution can scale its features appropriately and handle your enterprise workloads. Certain AIOps features that work well in lab or POC environments may struggle to keep up with larger-scale requirements typically encountered in production environments.
How can observability leverage deeper AI and machine learning beyond traditional definitions of AIOps?
It all starts with the data. The more comprehensive and rich the data that's available to analyze, the more that can be done with that data through the application of AI/ML technologies. Advanced application of AI/ML can help drive additional use cases such as extracting business insights, deriving predictive or leading indicators across multiple signals, or defining and deploying entirely customized AI/ML when the need arises.
An example of AI/ML use beyond traditional AIOps is natural language processing (NLP). NLP helps with categorizing and classifying textual data. NLP comes in handy for use cases like sentiment analysis that helps measure user sentiment tied to events like new application roll-out or major feature upgrades, complementing and enhancing observability.
For a tailor-fit solution to specific, custom enterprise requirements, IT teams can decide to bring their own ML modeling schemes to build, train, test, and deploy into production. The output from these models can be visualized in custom dashboards or reports, enabling IT organizations to cater to special, one-off, or specific needs not met through out-of-box capabilities.
What use cases and trends will AIOps be able to handle in the future?
As the data makes its journey from the point of collection, persistence (balancing data availability, performance with TCO), analytics, to visualization and remediation, AIOps will continue to play a significant part in that journey.
As data volumes continue to increase, AI-assisted analytics during data collection will help determine which data is most meaningful to collect and analyze. Running AI/ML algorithms at the collection edge will also aid in summarizing data at or close to the point of collection, distributing AI smarts and reducing the requirement to centralize all analytics. Classification and categorization schemes will dynamically decide whether incoming data is sent to warm or cold tiers for storage, optimizing TCO. Analytics will independently act on multiple signals and data, detecting anomalous behavior, then rapidly isolating the root cause. And remediation automation will become autonomous for more use cases and scenarios as customer trust starts to rise in auto remediation. Observability systems will start to form more closed loops; collecting, storing, and analyzing data, and detecting and remediating more incidents automatically with increasingly less human intervention.
AIOps matters for DevOps teams in today's cloud-native world
As with many newer technologies, the definition of AIOps and its benefits continues to evolve. Including AIOps as part of your observability strategy is part of the natural response to ever increasing data, complexity, and pace of change. And when implemented well, AIOps can drive significant business value.
Stay tuned as we take a deeper dive into common AIOps use cases for driving improvement and efficiencies in a future blog.