Using AIOps for automation and efficiency in observability and IT operations
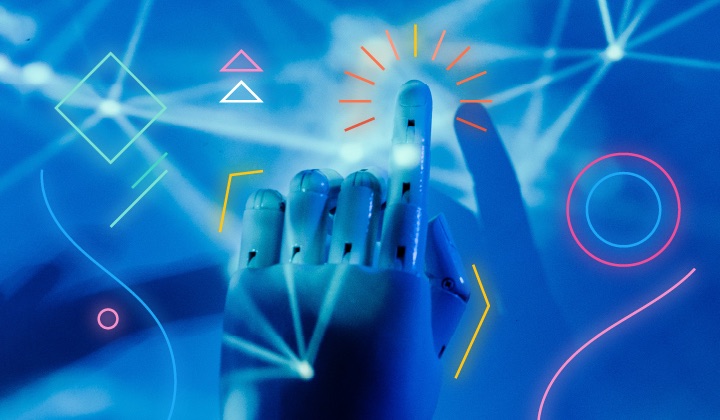
Artificial intelligence for IT Operations (or AIOps) has been playing an expanding role in helping SREs, DevOps, and developers effectively navigate the challenges around application and infrastructure complexity, pace of change, and data volume that characterize the operations landscape. By improving efficiency and effectiveness of application and infrastructure monitoring by automating many of the tasks, AIOps can help to reduce the workload on observability teams and free up time to spend on more meaningful analysis.
In our first post, What is AIOps? A beginner’s guide, we dove into AIOps: what it is, why it matters, and establishing production readiness. We talked about why it’s important for an AIOps initiative to start simple with time tested and proven AIOps capabilities, and then to slowly add more AIOps features as the benefits are realized and demonstrated.
AIOps can help reduce downtime and improve performance even further by using machine learning (ML) techniques to process and analyze large amounts of observability data (logs, traces, metrics, and related signals) to identify potential issues before they can cause disruptions. AIOps can also provide data-driven insights and predictive analytics to help organizations make more informed decisions about how to manage applications and infrastructure to optimize operations and maximize resources.
Practical AIOps use cases for observability
Let’s dive into some observability use cases and the AIOps capabilities that can help address them by automating common application and infrastructure monitoring tasks to gain better control.

AIOps for real-time monitoring
Observability platforms can ingest and analyze massive amounts of data from multiple sources in real-time, allowing SREs to get a comprehensive view of the system's behavior and identify potential issues as they arise. AIOps functions can be used to automatically identify patterns in the diverse data and highlight relationships and correlations that are not easily evident through common data visualizations and dashboards.
This can be particularly useful for detecting and resolving problems that are difficult to predict or that may be hidden within the system's normal operating range. For example, when an application is running slow, AIOps can be used to automatically identify probable causes of slow or failed transactions.
A search toolkit for the AI era
The Elasticsearch Relevance Engine (ESRE) gives developers the tools they need to build AI-powered search apps.
Build Generative AI Search Engines and ApplicationsAIOps for anomaly detection
AIOps can also be used to identify unusual behavior in the system, which can indicate a potential issue. By continuously analyzing data from various sources, AIOps platforms can detect deviations from normal patterns and alert SREs to the presence of anomalies. This can help SREs proactively identify and address issues before they cause major disruptions or impact application performance.
By automating the analysis of vast amounts of data from various sources (such as logs, metrics, and events), AIOps can help IT teams quickly and accurately identify and resolve issues, as well as optimize the performance and availability of the system.
AIOps for alert correlation and triage
With so much data being generated by modern systems, it can be overwhelming for SREs to sift through all the noise and determine which alerts are the most important. Observability platforms can use AIOps techniques and machine learning algorithms to identify patterns and correlations between different alerts, allowing SREs to prioritize their efforts and focus on the most pressing issues. There are many types of noisy data that can be reduced by AIOps automation, for example:
- Multiple sets of similar or duplicate information
- Too many detected issues and alerts (both manual and automatic), some of which might have the same underlying root cause
- Informational notification events
These all contribute to varying levels of noise in the observability data and workflows. Alert fatigue has never been more obvious for SRE or IT Operations teams in modern application deployments.
AIOps plays a significant part in reducing noise and surfacing the important insights with the right context to enable IT Operations teams to become more efficient. Automatically determining and surfacing entity health puts the operational focus back on the applications, services, and infrastructure rather than on individual pieces of data. Accurate health scoring can take into account the extent and severity of anomalous behavior exhibited by these entities.
By automatically prioritizing entities and information based on business and user impact, AIOps helps focus on what’s most critical. AIOps can also help detect and de-duplicate information based on data characteristics and can cluster or group similar information, presenting them together, further reducing noise when troubleshooting. As new types of observability signals and data are ingested, time series baselining via unsupervised machine learning and anomaly detection significantly reduces the manual effort needed to monitor and track that data.
AIOps for root cause analysis
When an issue does arise, AIOps can help SREs identify the root cause more quickly. By analyzing data from multiple sources, AIOps platforms can identify the underlying cause of a problem, even if it is not immediately apparent. This can help SREs resolve issues more efficiently and prevent them from reoccurring in the future.
Automatically surfacing contextual information surrounding the issue helps speed up the investigation by presenting relevant information inline and in workflows. AIOps can correlate multiple events and behaviors around an issue, aiding more holistic investigations and cutting down MTTD (mean time to detection) and MTTR (mean time to resolution). An example of such correlation and root cause analysis is the ability to surface attributes of the data that are disproportionately represented in the issue or anomalous event. One or more of those attributes can in turn point to the potential root cause. For a smaller set of specific, well understood symptoms, AIOps can fully automate the journey from symptom to root cause, removing the need to manually iterate through the investigation.
AIOps makes observability easier for operations and business
AIOps aims to ease the lives of IT operations teams, reducing the amount of manual work needed, especially for routine and repetitive tasks, and helps find the needle in the haystack. This allows operations users to focus on higher-level activities like platform architecture, platform engineering, automation, security, and more.
Modern hybrid and cloud-native environments continue to push the boundaries of what operations folks can manage for their enterprise. Cost analytics and tracking, business metrics, and alignment of business impact with observability data are just a few of the examples of the more recent challenges facing ops teams. The good news is that the same AIOps concepts and analytics capabilities such as baselining, anomaly detection, and correlations that help observability are equally adept at solving the newer business challenges. AI and ML capabilities can go even further and help make sense of any generic signals and data, allowing users to extract useful, actionable insights that contribute to business success. But that's a blog post for another day!
Read this next: What is AIOps? A beginner's guide, or watch this webinar: The impact of AIOps and GAI on modern observability.