Built-in protections for Elastic AI Assistant
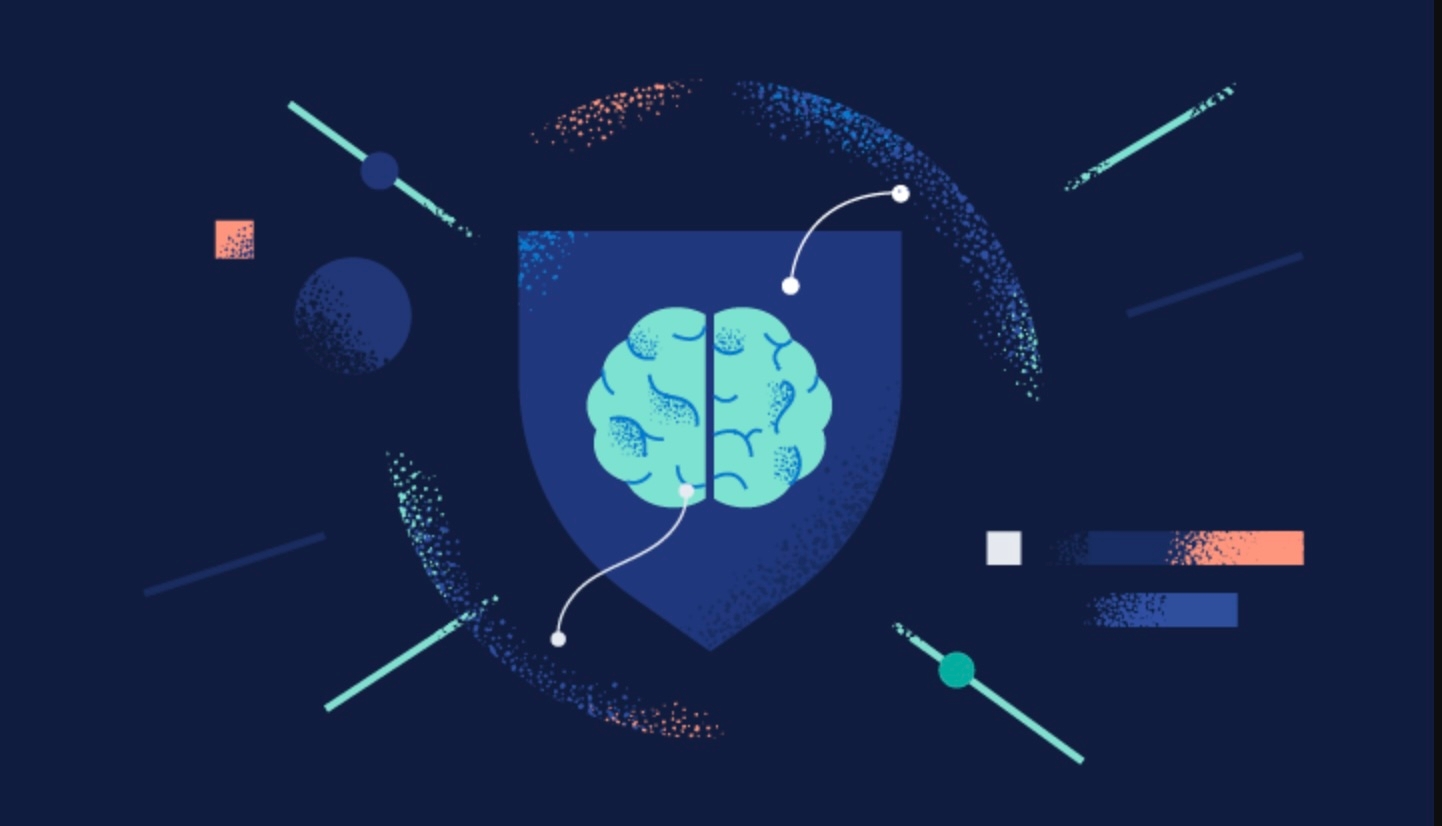
The rise of generative AI systems — including large language models (LLMs) — has ushered in a new era of possibilities, but it has also introduced novel security challenges. As these new systems become more prevalent, understanding and mitigating the associated risks is paramount. In its mission to democratize knowledge, Elastic Security Labs has released a brand new report: The LLM Safety Assessment.
This publication walks through important LLM implementation risks and threats, including research from the Open Worldwide Application Security Project (OWASP) on the ten most common techniques that threat actors utilize against LLMs. This blog will expand upon some of those techniques in relation to how Elastic's AI Assistant and Attack Discovery are designed to address these concerns.
Prompt injection (LLM01) and insecure output handling (LLM02)
Prompt injection exploits the model's dependency on input prompts to generate responses, potentially manipulating outputs to serve malicious objectives. Connected to this is insecure output handling, which refers to generative AI systems generating unsafe, biased, or inappropriate content.
To combat prompt injection and insecure output handling, Elastic’s AI Assistant provides a comprehensive history of persisted chats and LLM logs — enabling organizations to leverage Elastic Security’s built-in capabilities like the detection engine, machine learning, and more to monitor and alert on these threat techniques. By closely monitoring user inputs, model interactions, and generated outputs, security teams can detect potential prompt injection attacks as well as identify and mitigate insecure or harmful outputs.
Training data poisoning (LLM03)
This technique happens when malicious data is introduced into the model’s training set, potentially skewing outcomes or reducing the effectiveness of the AI. Elastic AI Assistant’s Knowledge Base feature supplements the training data with verified, up-to-date information, enhancing model accuracy and reducing dependency on unreliable data sources.
Supply chain vulnerability (LLM05)
Supply chain vulnerability attacks insert malicious elements into the AI's development pipeline, compromising the model before deployment. The AI Assistant permits seamless integration with a variety of LLMs, both cloud-hosted (such as OpenAI's GPT models and Amazon's Bedrock) and locally hosted (like Mistral and Llama). This flexibility ensures that organizations are not locked into one LLM provider and can choose the best hosting option based on their security needs, significantly reducing the risks associated with supply chain vulnerabilities.
Sensitive information disclosure (LLM06)
This threat involves the unauthorized release of confidential information through model interactions. To prevent this technique, the AI Assistant includes sophisticated anonymization capabilities. It provides customizable field-level options for managing structured context data, such as security alerts. Organizations can enforce stringent anonymization policies to selectively include or exclude specific fields and anonymize their values before they get processed by an LLM.
Anonymization is particularly crucial for alerts that contain personal identifiers or confidential data, ensuring these details are obscured to maintain privacy and compliance. By employing this capability, the AI Assistant not only secures data from unauthorized disclosure but also mitigates the risks of data leakage. This proactive approach supports the secure application of AI technologies in sensitive environments, safeguarding user privacy and enhancing overall system security.
Overreliance (LLM09)
Users may overly trust the accuracy of AI-generated responses, which can lead to misguided decisions based on inaccurate data. This is addressed through the Elasticsearch Relevance Engine (ESRE). The AI Assistant for Security can ground its answers in data unique to an organization, providing context that enhances the relevance and security of responses. For instance, if an organization's security team inquires about a specific threat indicator, the AI Assistant can leverage ESRE to provide a tailored response based on the organization's threat intelligence data. This feature improves performance while addressing the need for context to provide specific, applicable answers. This also prevents hallucinations — when a model accidentally makes up an answer — or misuse of generalized, publicly trained models.
Additional security measure: tokenization
Token tracking is a critical component of managing and securing interactions between users and LLMs using the AI Assistant for Security. At its core, tokenization refers to the process of converting data, such as text, into a sequence of tokens. These tokens serve as the basic unit of data that LLMs analyze to understand and generate human-like responses. Each token can represent a word, part of a word, or even punctuation, making them fundamental to the way LLMs process and comprehend input data.
Adopting generative AI securely and responsibly
By proactively addressing these LLM threats, Elastic's AI Assistant for Security represents a comprehensive and forward-thinking approach to securing generative AI systems. From custom rules and alerts on LLM logs to integration with a variety of different providers and Elasticsearch Relevance Engine (ESRE), Elastic has implemented a robust set of security features to ensure the safe and responsible deployment of LLMs.
As the AI landscape continues to evolve, Elastic remains committed to staying ahead of emerging threats and incorporating the latest security best practices into its AI Assistant for Security. By prioritizing ethical responsibility and data protection, Elastic paves the way for the secure adoption of generative AI technologies across industries. You can read more on our suggested best practices for LLM threats with the new LLM Safety Assessment.
The release and timing of any features or functionality described in this post remain at Elastic's sole discretion. Any features or functionality not currently available may not be delivered on time or at all.
In this blog post, we may have used or referred to third party generative AI tools, which are owned and operated by their respective owners. Elastic does not have any control over the third party tools and we have no responsibility or liability for their content, operation or use, nor for any loss or damage that may arise from your use of such tools. Please exercise caution when using AI tools with personal, sensitive or confidential information. Any data you submit may be used for AI training or other purposes. There is no guarantee that information you provide will be kept secure or confidential. You should familiarize yourself with the privacy practices and terms of use of any generative AI tools prior to use.
Elastic, Elasticsearch, ESRE, Elasticsearch Relevance Engine and associated marks are trademarks, logos or registered trademarks of Elasticsearch N.V. in the United States and other countries. All other company and product names are trademarks, logos or registered trademarks of their respective owners.