On-demand webinar
The Math Behind Elastic Machine Learning
Hosted by:
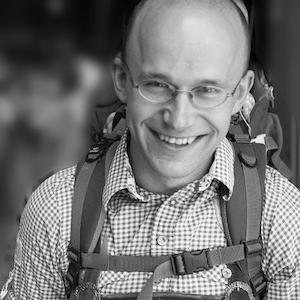
Hendrik Muhs
Software Engineer
Elastic
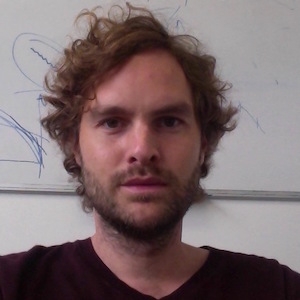
Tom Veasey
Principal Software Engineer
Elastic
Overview
Machine learning functionality is being added to our products in the form of shrink-wrapped applications. We began by focusing on time series anomaly detection, which required the ability to predict what the time series might do next. It wasn't such a large step to go from this to forecasting what the time series might do over the next day, week, or month. This capability was added in 6.2.
To automate this process, an ML system needs expressive modeling, which can adapt to different data characteristics, relearn its parameters (or select a new model as things change), seamlessly deal with abnormal periods to minimize their impact on the modeling, and much more. Dig into the details of some of the modeling techniques we’ve used for these features and some of the key ways we have addressed these requirements.
Register to watch
You'll also receive an email with related content.
MarketoFEForm