Relevance workbench
In this workbench, you can compare our Elastic Learned Sparse Encoder model (with or without RRF) and traditional textual search using BM25.
Start comparing different hybrid search techniques using TMDB’s movies dataset as sample data. Or fork the code and ingest your own data to try it on your own!
Try these queries to get started:
- “The matrix”
- “Movies in Space”
- “Superhero animated movies”
Notice how some queries work great for both search techniques. For example, 'The Matrix' performs well with both models. However, for queries like "Superhero animated movies", the Elastic Learned Sparse Encoder model outperforms BM25. This can be attributed to the semantic search capabilities of the model.
Explore similar demos
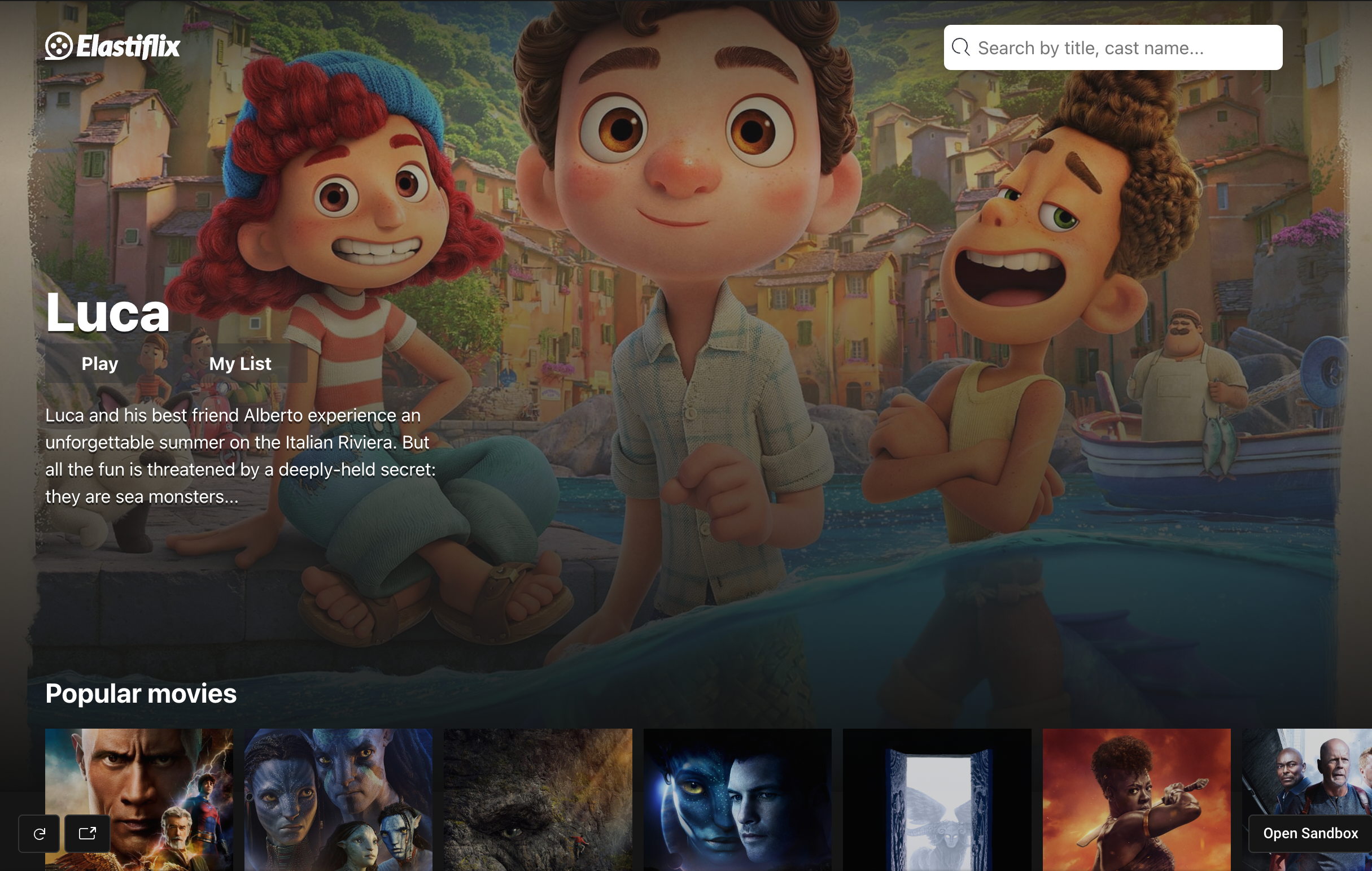
Search
Movie search application
Try out this live demo of a movie search application built on top of Elastic Search.
The first part is a front end application implemented using Search UI. Feel free to just play with it or clone the code and try yourself!
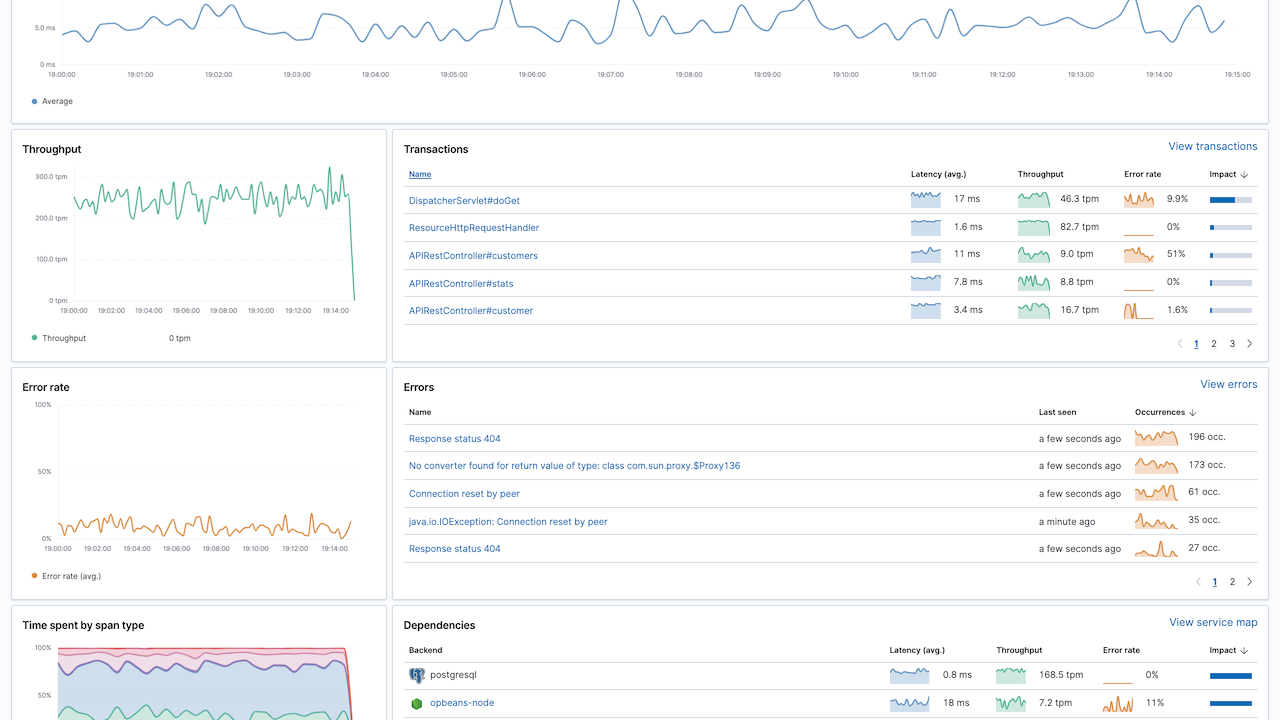
Observability
APM - Java
Already housing logs and system metrics in Elasticsearch? Expand to application metrics with free and open Elastic APM. See exactly where your application is spending time so you can quickly fix issues and feel good about the code you push.
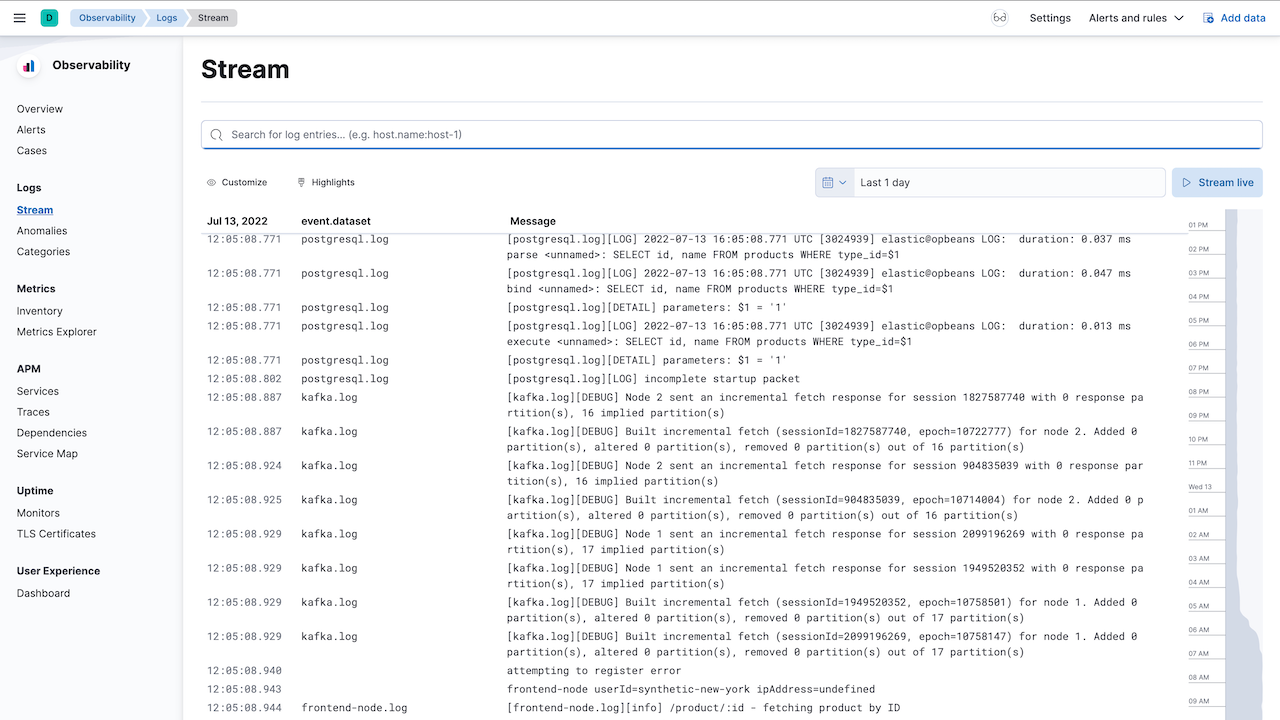
Observability
Logging
Keep a pulse of all log files flowing in from your servers, virtual machines, and containers in a purpose-built and intuitive interface for viewing logs. Pin structured fields and explore related logs without leaving your current screen. Dive into your real-time streaming logs in Kibana for a console-like experience.