On-demand webinar
Time Series Anomaly Detection: Optimizing your Machine Learning Jobs in Elasticsearch
Hosted by
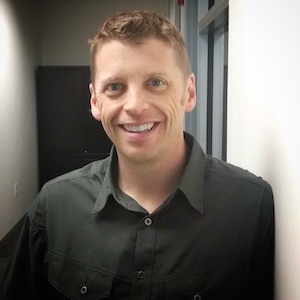
Rich Collier
Solutions Architect, Machine Learning Specialist
Elastic
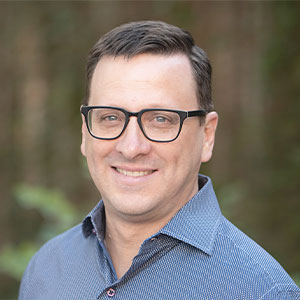
Tom Grabowski
Principal Product Manager
Elastic
Overview
Got logs and metrics (or any time stamped data really) in Elasticsearch, and looking to craft your 1st machine learning job to automate anomaly detection? Watch a practical walkthrough on efficiently progressing your machine learning projects from concept to production.
Elastic Machine Learning experts kick off the presentation with the Elasticsearch and machine learning basics, and then demomstrate the iterative process of crafting and optimizing your machine learning jobs.
Highlights include:
- Leveraging Kibana visualization tools to build intuition about the data
- Using scheduled events to exclude expected anomalies from data / model
- Forecasting into the future using the trained model
- Getting proactive via alerting integrations
Additional Resources (Related content)
Register to watch
You'll also receive an email with related content.